Click-on-Ads
Goal
Provide support in prioritizing the target audience to enhance ad conversion rates and drive improvements in marketing campaign performance.
Result
A reliable predictive framework that effectively identifies prospects with the highest conversion potential, optimizing budget allocation and minimizing overspending in less promising audiences.
Case
As the advertising landscape becomes increasingly data-driven, it's crucial to develop predictive click-on-ads models to optimize targeting and achieve cost-effective advertising strategies, boosting conversions and delivering personalized messaging for higher click-through rates.
Are There any Insights to be Gained from Examining the Distribution of Age in the Dataset?
A notable trend in the dataset is the concentration of potential customers between the ages of 25-35, with individuals in their late 20s to early 30s showing the highest level of engagement on the website.
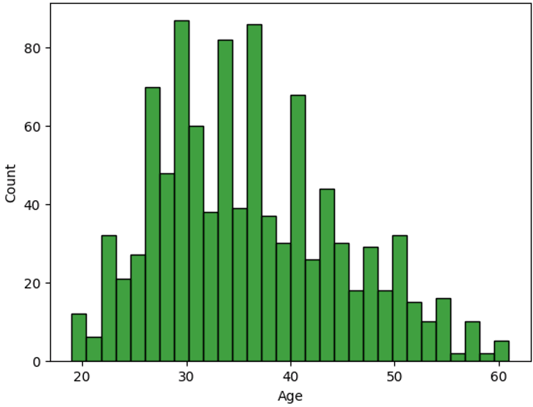
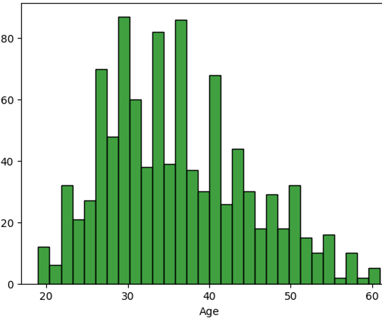
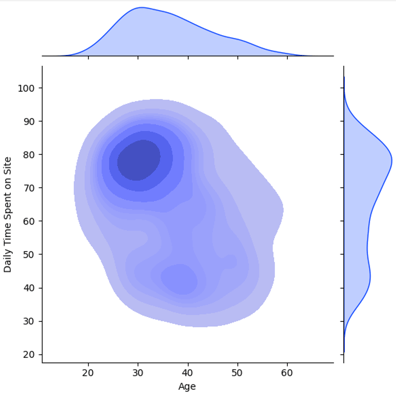
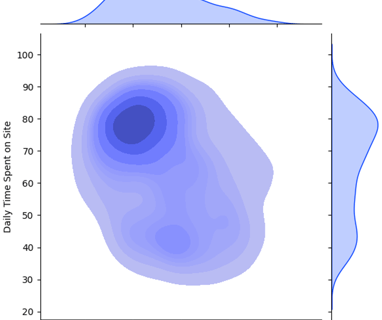
Are There any Notable Patterns Worth Exploring?
Yes, three:
It's striking to find a strong inverse correlation between daily internet usage and conversion rate.
Contrary to expectations, increased time spent on the site is associated with a lower probability of clicking on ads.
Age shows a robust positive correlation with click rates, suggesting that older individuals are more likely to click on ads.
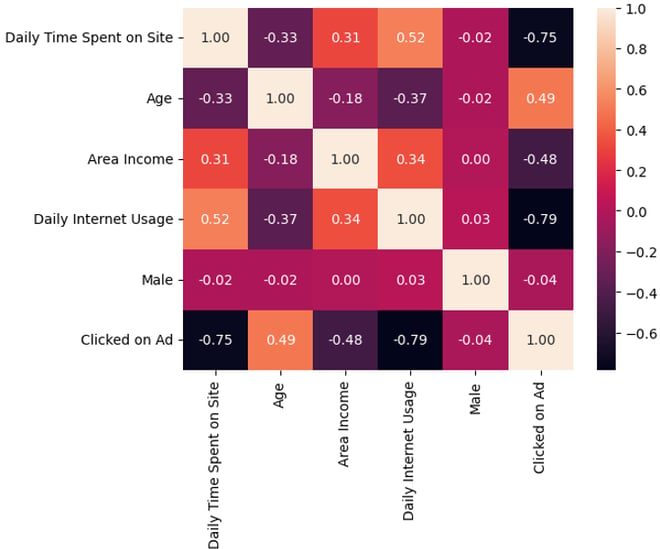
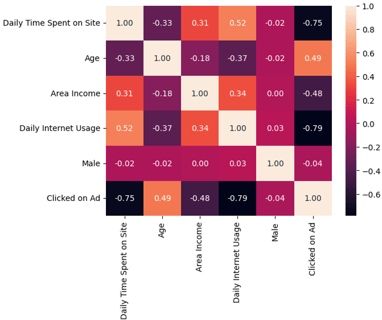
Which is the Best Prediction Model?
Following an extensive evaluation of different models, it has been established that the Naïve Bayes classifier stands out as the most effective choice for this task -perhaps for the number of samples-, achieving exceptional metrics such as accuracy (0.96), AUC (0.99), F1 score (0.96), Kappa (0.93), and Matthew's Correlation Coefficient (0.93).
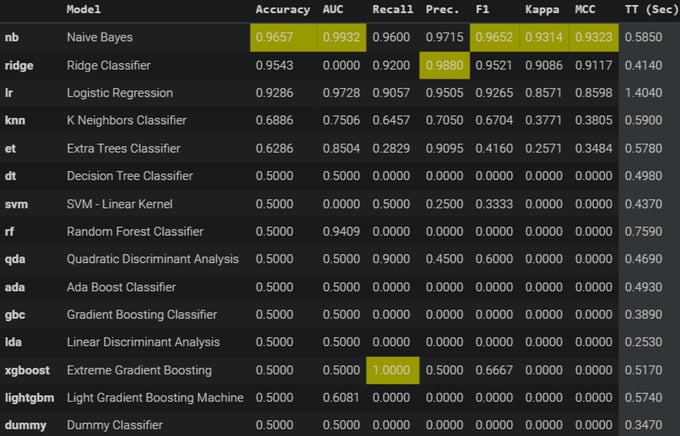
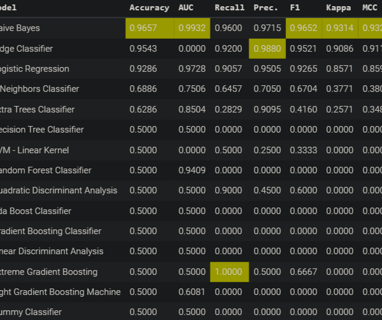
How Robust is the Model?
Upon applying imputation, target encoding, and data cleaning techniques, we observe a substantial improvement in both training score and cross-validation score, with both values nearing 0.97, not having a considerable variability.

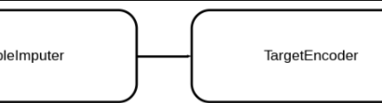
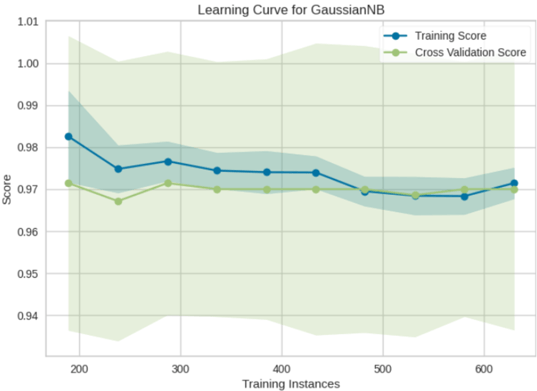
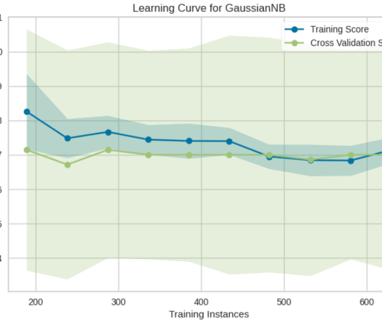