Customer Churn Prediction
Goal
A key priority for the company is to execute tailored retention strategies and interventions that effectively manage the customer lifecycle. By doing so, the aim is to enhance customer retention rates, maximize customer lifetime value, and elevate overall business performance.
Result
Foster greater customer loyalty and value by implementing an effective decision-making framework that boosts customer retention efforts and positively impacts customer base health.
Case
Building a customer churn prediction model offers numerous benefits. It leads to cost reduction by prioritizing resources towards retaining at-risk customers rather than expensive acquisition efforts. This not only increases the chances of retaining customers but also enhances customer satisfaction.
Overall, investing in a customer churn prediction model yields significant advantages in customer retention, cost savings, marketing effectiveness, competitive positioning, and revenue generation.
How are Churn and Age Related?
The data suggests that individuals aged 27-28 are more susceptible to churning, while those aged 30-31 exhibit a lower susceptibility to churn. This pattern highlights the significance of age as a predictive variable for churn prediction.
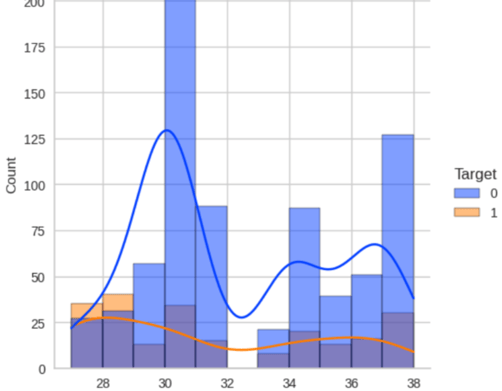
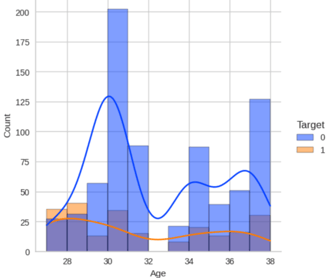
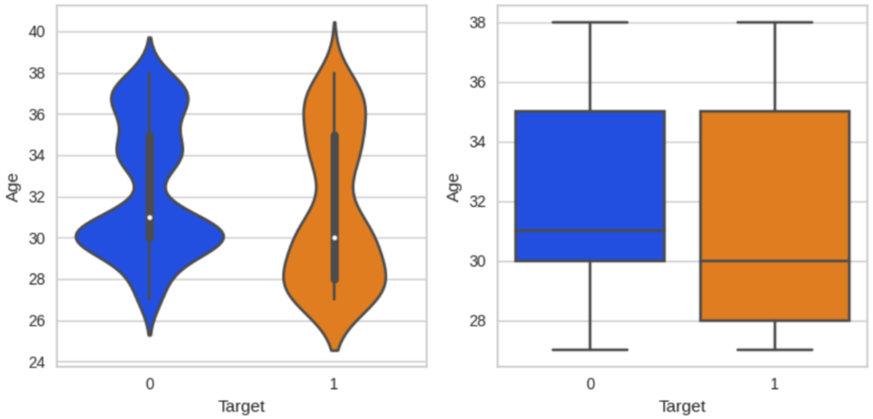
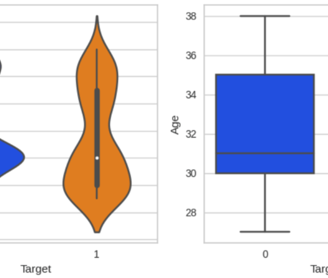
What important patterns do the variables show?
There are three interesting patterns:
The data reveals a significant inverse correlation between annual income and customer churn, indicating that higher-income individuals are more prone to churning.
Frequent flyers show the highest likelihood of churn, suggesting a strong association between frequent travel and customer churn.
A notable trend emerges, indicating that customers who have booked hotels tend to exhibit higher levels of loyalty and are less likely to churn.
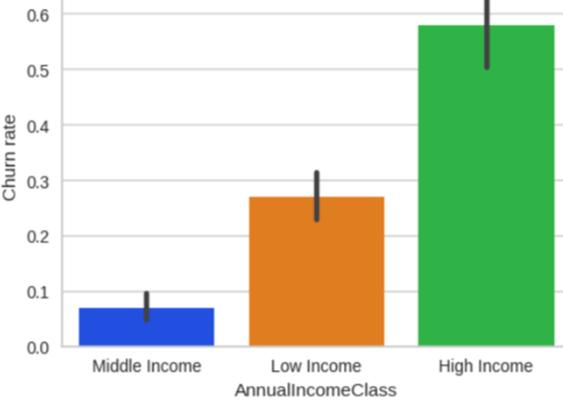
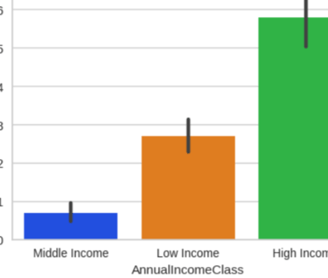
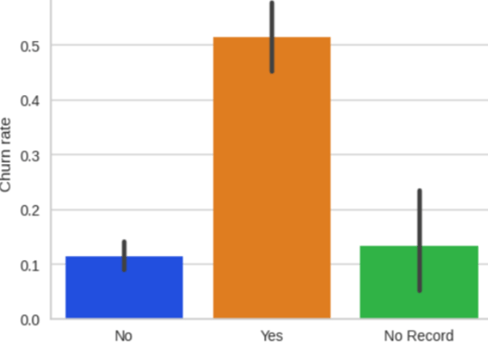
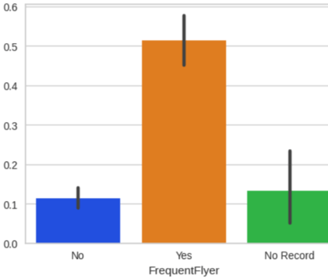
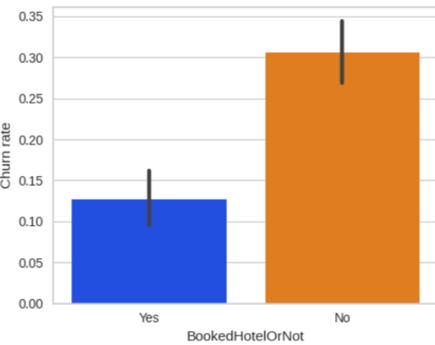
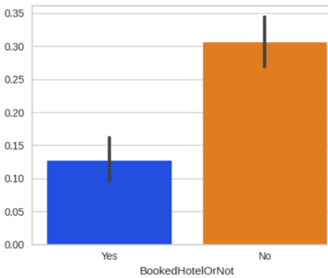
Which is the Best Model?
LGBM outperforms other models in terms of accuracy (0.87), recall (0.73), F1 score (0.72), Kappa coefficient (0.64), and Matthew's correlation coefficient (0.64), showcasing its robust performance.
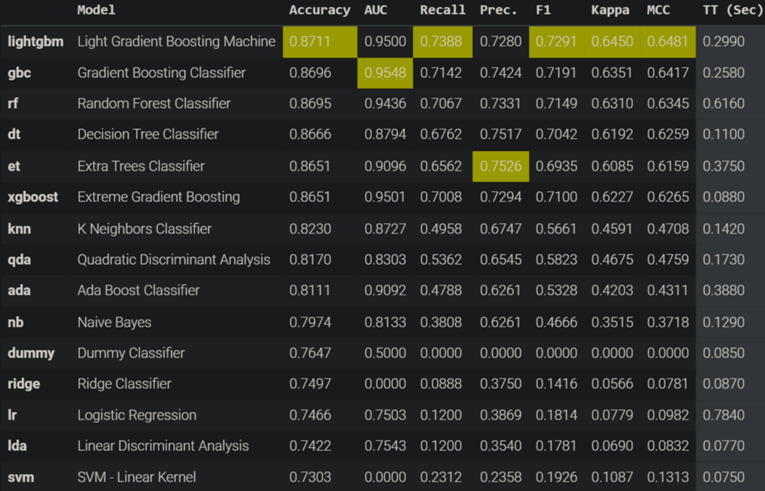
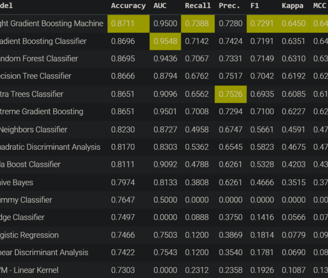
How Robust is the Model?
With a remarkable AUC score of 0.96, the LGBM model demonstrates superior classification ability, and the marginal difference between the training score (0.95) and cross-validation score (0.875) suggests consistent performance across datasets..
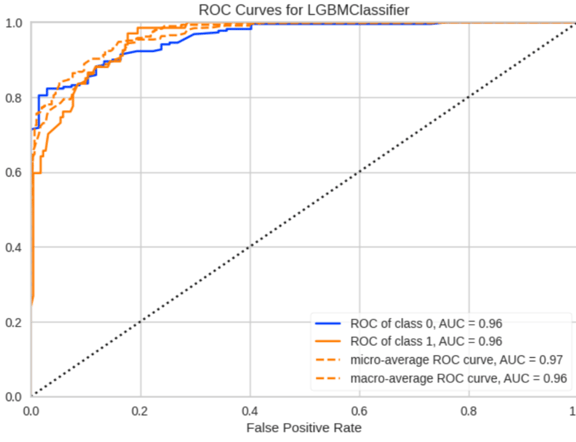
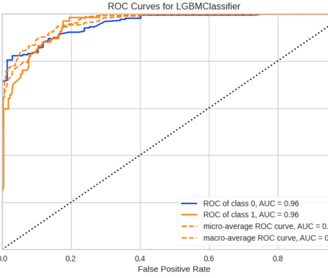
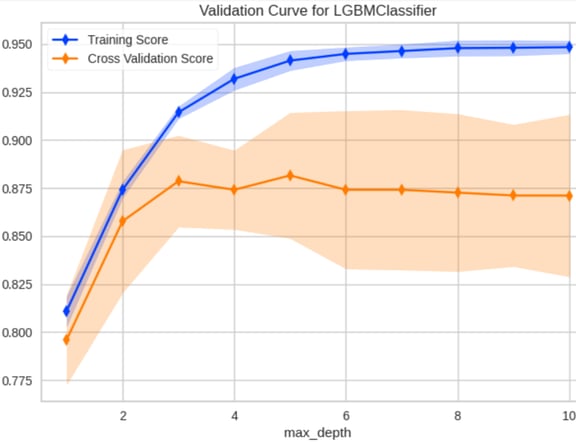
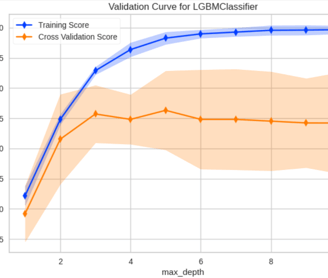
What insights can be inferred?
Three important insights:
The age variable emerges as a critical factor in the model, emphasizing the need to prioritize preventive retention efforts within the age groups at the highest risk.
The choices and preferences regarding service quantity and type present a significant avenue for fostering customer loyalty and should be leveraged as a potent opportunity.
To effectively retain customers, it is essential to segment retention efforts based on income levels, recognizing the varying needs and expectations of different income groups..
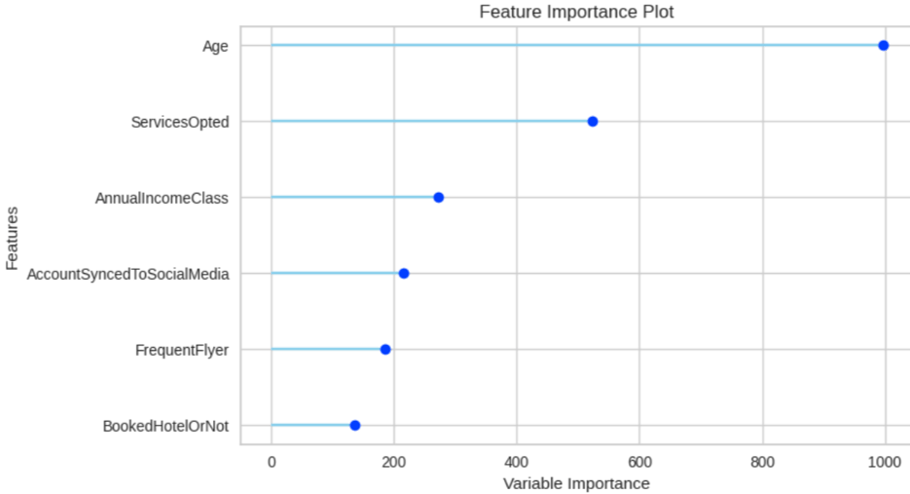
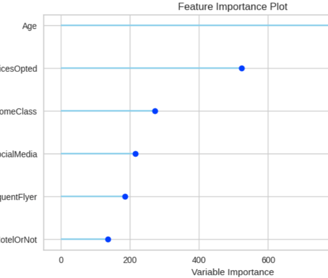